Antibiotic resistance is one of the most serious and silent health threats of our time. In hospitals—where vulnerable patients and complex infections are concentrated—the presence of antibiotic-resistant bacterial strains can severely undermine treatment effectiveness, prolong hospital stays, and increase the risk of complications and mortality. For this reason, predicting antimicrobial resistance is of crucial importance.
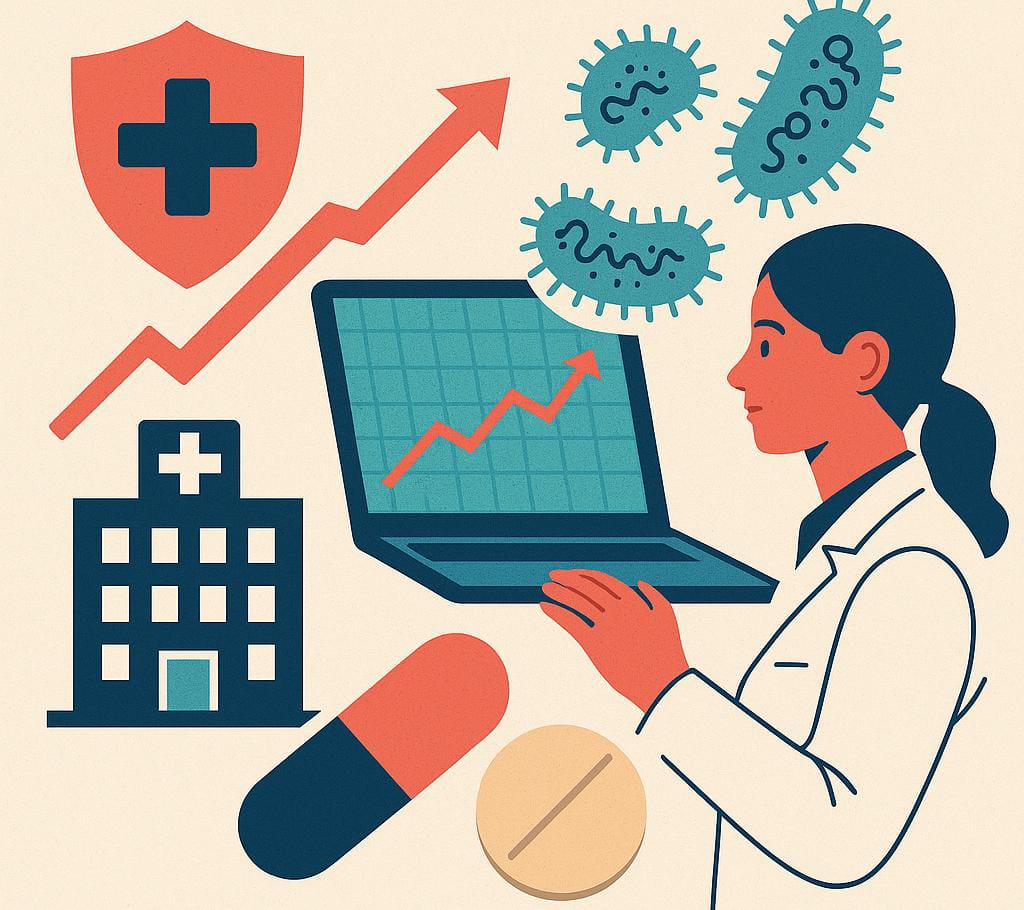
What Is Antibiotic Resistance?
Antimicrobials – including antibiotics, antivirals, antifungals, and antiparasitics – are medicines used to prevent and treat infectious diseases in humans, animals and plants. Antimicrobial Resistance (AMR) occurs when bacteria, viruses, fungi and parasites no longer respond to antimicrobial medicines. As a result of drug resistance, antibiotics and other antimicrobial medicines become ineffective and infections become difficult or impossible to treat, increasing the risk of disease spread, severe illness, disability and death [1] .
This phenomenon, accelerated by the overuse and misuse of antibiotics in both human and veterinary medicine, has made many common infections harder—or even impossible—to treat. In hospital settings, this issue is especially critical. Patients are often immunocompromised or recovering from invasive procedures, creating ideal conditions for resistant infections to spread rapidly.
The Importance of Prediction
Predicting antibiotic resistance is emerging as one of the most promising strategies to tackle the problem. This isn’t about guesswork—it involves the use of mathematical models and predictive algorithms that analyze clinical, microbiological, and epidemiological data to anticipate resistance trends [2].
Being able to effectively predict antibiotic resistance offers several critical advantages. First and foremost, it helps avoid the use of ineffective treatments, which in turn reduces the misuse and overuse of antibiotics—a major factor in the rise of resistance. It also allows healthcare providers to respond more quickly to outbreaks of resistant infections, containing their spread before they become unmanageable. Another key benefit is the ability to personalize therapies. By understanding the specific characteristics of both the patient and the infecting pathogen, doctors can choose treatments that are more likely to be effective from the start. Finally, prediction supports better overall hospital management. With accurate data and forecasts, hospitals can implement smarter prevention and infection control strategies, making care more efficient and safer for everyone.
Data and Models: A Cross-Disciplinary Effort
At the heart of this approach lie mathematical models and AI tools capable of analyzing vast amounts of data—from bacterial genomic sequences to clinical case statistics and environmental information from hospital wards. These tools can identify hidden patterns and critical correlations that would be hard to spot through traditional analysis [3].
Building and refining such systems requires collaboration across disciplines: engineers, physicians, microbiologists, physicists, and computer scientists all contribute. It’s a powerful example of how interdisciplinary work is essential in addressing complex challenges like antibiotic resistance.
Looking Ahead
While antibiotic resistance is a natural and partially unavoidable process, its impact can be mitigated through targeted prevention, monitoring, and response strategies. Predicting antimicrobial resistance plays a key role in this effort—it offers a way to gain time, optimize treatment, and ultimately save lives.
Investing in research, predictive technologies, and interdisciplinary collaboration is no longer optional—it’s necessary. In the fight against resistant bacteria, every well-timed decision makes a difference.